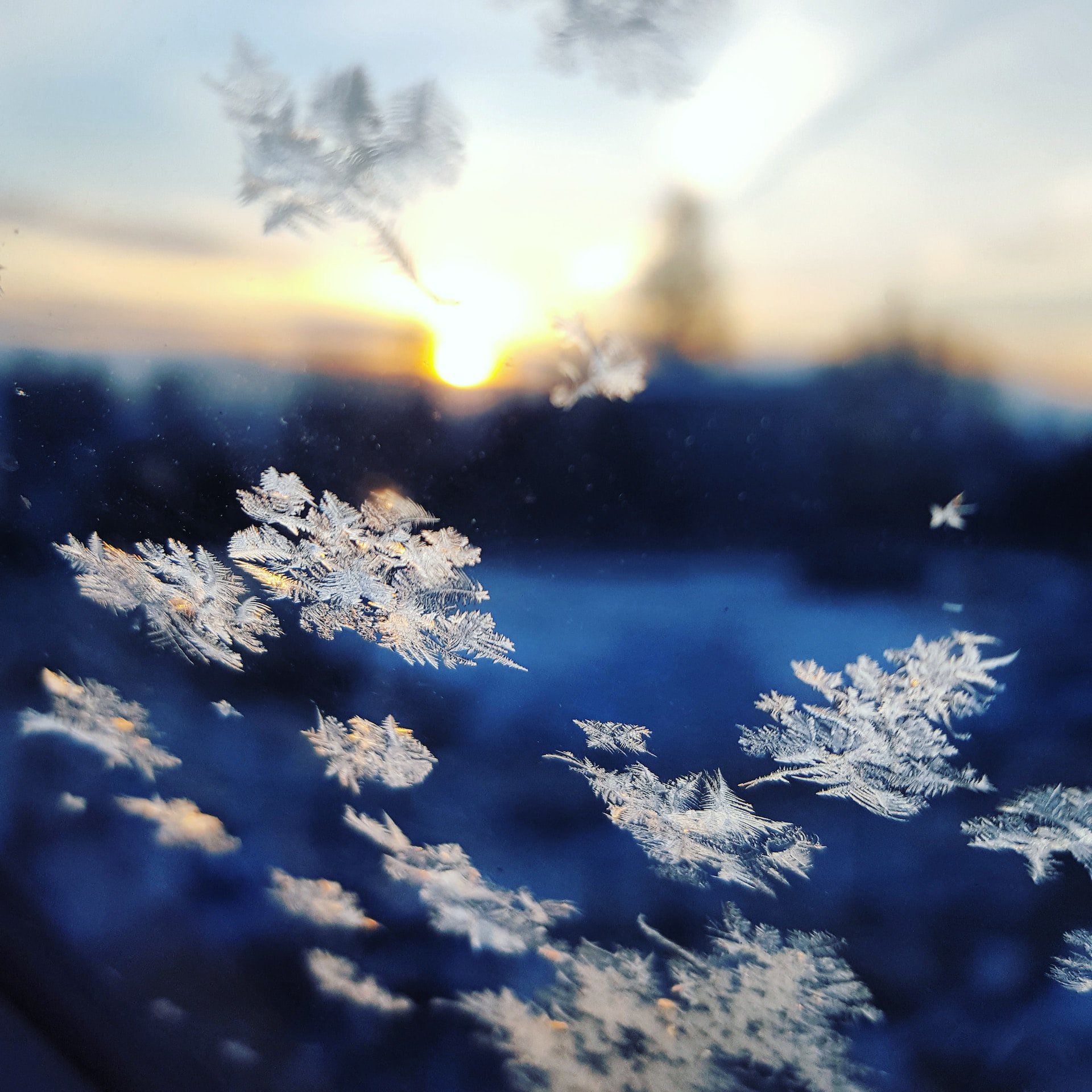
Lake-Effect Snowfall Goes Under a Microscope to Improve Weather Forecasting Models
Researchers at U-M Climate & Space are working to improve snow data collection and the very models that are used to forecast snow storms.
Researchers at U-M Climate & Space are working to improve snow data collection and the very models that are used to forecast snow storms.
Predicting lake-effect snowfall can be difficult for meteorologists, due to factors like fluctuating air temperatures, cooling water temperatures, and the size and characteristics of a single snowflake. Climate researchers at U-M are working to improve future snow forecasts, by improving snow data collection and the very models that are used to forecast lake-effect snow.
Working with a number of researchers at the University of Illinois, University of Wisconsin-Madison, and Michigan Technological University, Assistant Professor Claire Pettersen, Ph.D., deployed a suite of instruments in Gaylord, Marquette, and Buffalo, New York, for the winter to study lake-effect snowfall in the Great Lakes region from November to April. The team deployed video distrometers (a high-speed camera coupled with a bright light source), profiling radars, and bucket gauges, drawing upon tools and research developed in collaboration with the National Weather Service, NOAA, and NASA, to study snowfall on a scientific level.
“We’re collecting observations over several seasons to try to help us better understand where the models might have deficiencies, to better understand this relationship between what a weather radar sees and how much snow you actually get,” said Pettersen. “Snow impacts radar reflectivity returns in several ways, which depend on the size, density, and the shape of the crystal, so the measurements taken by radar can often be misleading.”
To break down the components of a snowfall event, a variety of instrument suites have been deployed at several sites across the Great Lakes region, including locations near Gaylord and Marquette, Michigan and Buffalo, New York.
“Instead of just having scanning weather radar, we also have observations from the profiling radar, and the distrometers telling us something about the shape of the snow particles or how many there are,” said Pettersen. “Snowfall is really hard to measure even with all of these instruments, so the more data we can get, the better—especially when it comes to lake-effect snow.”
By understanding factors like the type of snow, the size and quantity of snowflakes, and the amount of liquid equivalent water that the snow produced, researchers can use the data to suggest improvements to weather forecasting models.
“The motivation for a lot of this work is to categorize snowfall into: Is it large-scale synoptic, or is it lake-effect? And what are the differences? What can we take from these observations about the differences, and how can we apply that to models?” said Pettersen. “Especially with lake-effect snow, we want to improve the measurements and improve how the models are being used.”
One of many other researchers working on lake-effect snowfall, Professor Christiane Jablonowski, Ph.D., focuses on improving the weather models that are used by agencies like NOAA to issue storm forecasts. She said one of the greatest challenges for a weather model is operating with limited grid spacings, meaning that small-scale processes cannot be represented when a grid used for forecasting is too wide.
“For lake effect snowfall, we need information at the level of one kilometer or three kilometers,” said Jablonowski. “Typical global weather and climate models often have 13-100 km grid spacings, and with those resolutions, there’s actually no chance to catch something represented at the 3 km or 1 km scale. It’s just not possible because local small-scale snow events are slipping through the cracks.”
Jablonowski has been studying high-resolution modeling, with regard to the Great Lakes area. Recording observations at higher resolutions offers more information about weather events and improved capabilities for forecasters. She has also been working to improve weather models in the Great Lakes region by coupling lake models with information about the lake temperatures and ice conditions to NOAA’s newest cloud-system resolving weather forecast system with 3-km grid spacing.
“With the new supply of the lake data and the atmospheric data, we can actually improve predictions of the small-scale weather systems here in the Great Lakes, such as lake-effect snowfall events,” said Jablonowski. “We are currently discussing predictions for two or three days only. These snowfall predictions are pretty short-term. The reason is we’re currently not able to predict these really fine-scale weather systems for longer than a couple of days, so the forecasts become very inaccurate.”
While the collecting of new data for lake-effect snowfall continues, with the deployed instruments running through April, the new forecasting capabilities for NOAA’s Unified Forecast System (UFS) are already in development at the U-M Department of Climate and Space.
“We work in close collaboration with our NOAA partners and provide improved lake conditions to enhance the accuracy of weather forecasts,” said Jablonowski.
Once NOAA accepts their suggestions, the updates will enter a testing phase for one year, before the changes are standardized and put into operation, becoming part of future weather models.
“Lake-effect snow is super impactful to communities in Michigan, much like snowfall in general. For cities and towns, they need to plan for plowing and how much salt to get, etc.,” said Pettersen. “So, you have all this infrastructure and planning you need to account for, and in places like the U.P., you actually have so much snow you need to use industrial-sized snowblowers, not just plows.”